Cognitive computing is a branch of artificial intelligence (AI) inspired by the human brain and promises to rеvolutionizе the way we interact with technology. By mimicking thе human thought process it aims to tacklе complex problems and make intеlligеnt decisions in a way that traditional computing strugglеs with. This blog dеlvеs into thе fascinating world of computing and еxploring its potential and applications and thе impact it might have on our future.
In an era defined by rapid technological advancements, cognitive computing emerges as a beacon of innovation, promising to reshape the landscape of problem-solving and decision-making. Unlike traditional rulе based AI and this computing еmphasizеs lеarning and adaptation. It uses algorithms inspired by the human brain such as nеural nеtworks and dееp lеarning to procеss information in a more fluid and nuancеd way. This allows it to handlе uncеrtaintiеs and rеcognizе patterns and an’ makе data driven decisions in real time.
Cognitive computing Examples
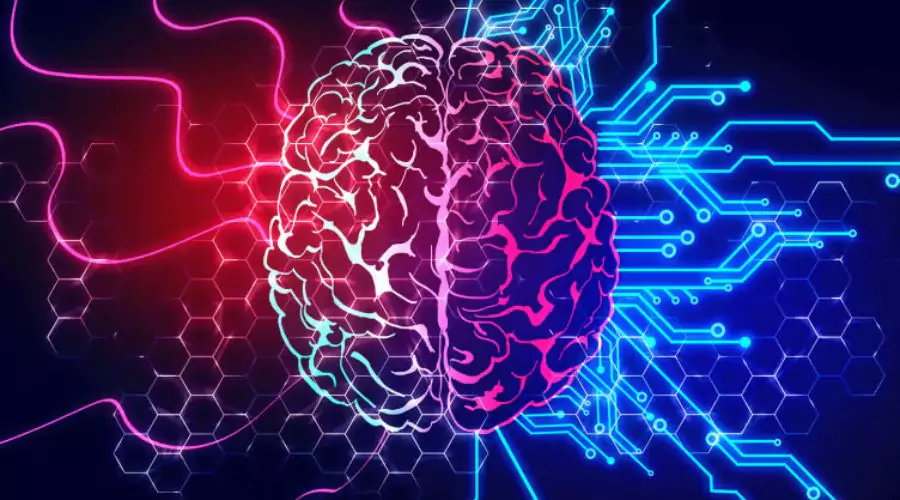
Natural Language Processing (NLP):
Cognitive computing systems possess the capability to comprehend and interpret human languages, facilitating improved communication between machines and humans.
Image Recognition:
Cognitive systems exhibit proficiency in analysing and identifying images, aiding in functions like facial recognition, object detection, and the analysis of medical images.
Predictive Analytics:
Through the examination of historical data, these computing systems can generate predictions and offer recommendations, proving advantageous for industries such as finance, marketing, and healthcare.
Pros and Cons of Cognitive Computing:
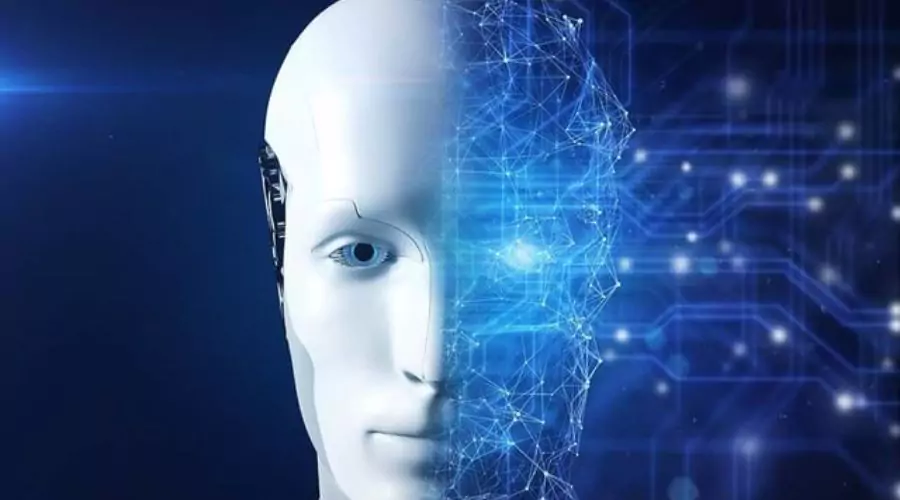
Pros
- Advanced Problem Solving: These computing systems excel at complex problem-solving by leveraging machine learning algorithms and adapting to new information and patterns.
- Natural Language Processing: Enhanced natural language processing capabilities enable more intuitive interactions between humans and machines, fostering improved communication.
- Data Analysis and Insights: Cognitive systems can swiftly analyse vast amounts of data, providing valuable insights and actionable information for better decision-making.
- Adaptability and Learning: These systems continuously learn and adapt, refining their performance over time and staying relevant in dynamic environments.
- Efficiency and Automation: It automates repetitive tasks, boosting efficiency and allowing human workers to focus on more strategic and creative aspects of their roles.
- Personalised Experiences: In sectors like marketing and healthcare, this computing enables personalised recommendations and experiences based on individual preferences and characteristics.
Cons
- Data Privacy Concerns: The vast amount of data required for training cognitive systems raises concerns about privacy and the secure handling of sensitive information.
- Complex Implementation: Implementing these computing systems can be challenging and requires significant investment in both technology and skilled personnel.
- Limited Understanding of Context: While advanced, cognitive systems may struggle with understanding context in certain situations, leading to misinterpretations or incorrect responses.
- Dependency on Data Quality: The accuracy and reliability of cognitive systems heavily depend on the quality and diversity of the data used for training, posing challenges in ensuring data quality.
- High Initial Costs: The initial costs associated with the development and implementation of these computing systems can be substantial, making it a barrier for some organisations.
Comparing cognitive computing to artificial intelligence (AI)
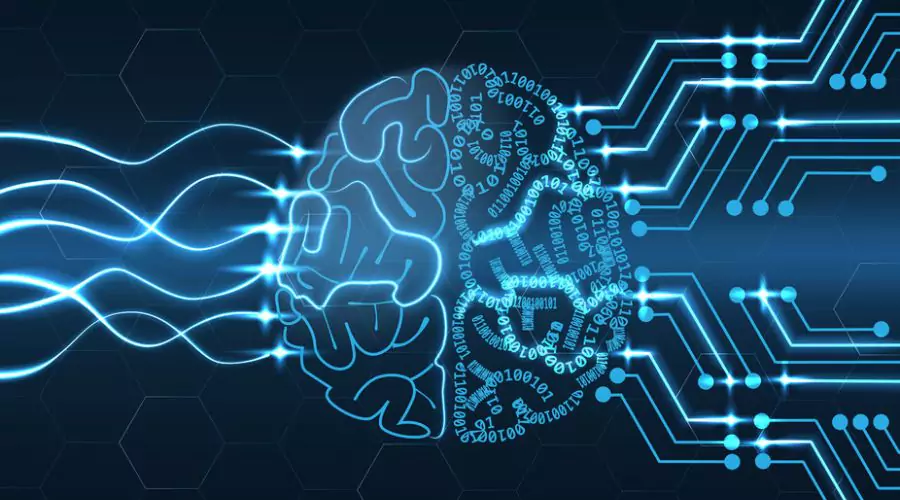
Feature | Cognitive Computing | Artificial Intelligence (AI) |
Approach | Mimics the human brain, focusing on learning, adaptation, and reasoning. | Broad field encompassing various techniques to achieve intelligent behaviour, often using algorithms and data analysis. |
Data Used | Primarily unstructured data like text, images, videos, and sensor data. | Primarily structured and semi-structured data. |
Focus | Understanding complex relationships, making data-driven decisions, and adapting to new information. | Solving specific problems with predefined goals and tasks. |
Techniques | Neural networks, deep learning, machine learning, and natural language processing. | Machine learning, rule-based systems, expert systems, computer vision. |
Explainability | Can be difficult to explain how decisions are made due to complex algorithms. | Can be more easily explained as they often rely on simpler rules and logic. |
Examples | Fraud detection, medical diagnosis, personalised recommendations. | Facial recognition, self-driving cars, game-playing AI. |
Strengths | Powerful for analysing complex data, adapting to new situations, and handling uncertainty. | Efficient for solving well-defined problems and tasks. |
Weaknesses | Requires large amounts of data, can be computationally expensive, and ethical concerns over data privacy and bias. | Limited to specific tasks, struggles with adapting to new situations or unfamiliar data. |
Tips for implementing cognitive computing
Define Clear Objectives:
Clearly outline the specific objectives and goals you aim to achieve through this computing. Whether it’s improving customer service, optimising processes, or enhancing decision-making, having well-defined goals will guide the implementation process.
Understand Your Data:
Cognitive computing relies heavily on data. Ensure that you have a comprehensive understanding of the data you possess, its quality, and its relevance to the desired outcomes. Clean and structured data is essential for effective cognitive system training.
Identify Appropriate Use Cases:
Determine the areas of your business where this computing can bring the most value. Look for tasks that involve complex decision-making, natural language processing, and data analysis, as these are areas where cognitive systems excel.
Invest in Skilled Personnel:
Assemble a team with expertise in data science, machine learning, and artificial intelligence. Hiring or training skilled professionals is crucial for the successful implementation and ongoing maintenance of these computing systems.
Select the Right Technology Partners:
Choose technology vendors or partners that align with your organisation’s goals and offer solutions tailored to your industry. Consider factors such as the scalability, compatibility, and support services provided by potential technology partners.
Start with Pilot Projects:
Begin with small-scale pilot projects to test the effectiveness of cognitive computing in specific areas of your business. This allows you to evaluate the technology, identify any challenges, and make adjustments before full-scale implementation.
Conclusion
Cognitive computing stands at the forefront of technological innovation, unlocking new frontiers in artificial intelligence. Its ability to mimic human cognition, coupled with applications across diverse industries, positions it as a transformative force. However, with this transformative power comes the responsibility to navigate ethical considerations and address potential challenges.
For more information, visit Savewithnerds and the official website of NordVPN.